Artificial Intelligence (AI) Software Toolkit
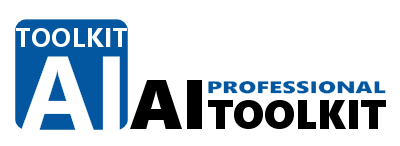
The AI-TOOLKIT is a software toolkit for building and using state of the art Machine Learning models (easy Training, Testing and Inference) and for building Intelligent Systems (several AI models working together). No programming skills are needed at all for building and using state of the art machine learning models!
The AI-TOOLKIT is compatible with MS Windows 64-bit 7, 8, 10 and above and requires a CPU released after 2012 (including the AVX processor extension). The software is optimized for Intel processors including the AVX processor extension but it will also work on other processors having the AVX extension. Most of the processors released after 2012 have the AVX extension (except some low cost laptop processors). The AI-TOOLKIT is hardware accelerated in several ways for maximum speed and performance. The number of processor cores is important, the more cores the faster the software will be! In case you intend to use the software professionally in your business then it is recommended to obtain a recent multi-processor core PC.
Easy to use and contains several types of machine learning models which can be used separately or connected to each other (ML Flow). There is also a built-in very fast SQL database to make the storage of your machine learning data compact and easy. The database supports several GB's of data storage and several databases can be used in a project.
What is included:
- AI-TOOLKIT Professional (flagship product)
- DeepAI Educational (educational deep neural network software with visualization of machine learning internals)
- The other AI-TOOLKIT products (VoiceBridge, VoiceData, DocumentSummary, etc.) are explained in their own web pages.
The AI-TOOLKIT also contains several easy to use professional applications:
- Face Recognition in images.
- Speaker Recognition in voice recordings.
- Fingerprint Recognition in images.
- Image Editor.
- Audio Editor.
- Big Text Files Editor.
- etc.
The AI-TOOLKIT supports the three major forms of machine learning: supervised, unsupervised and reinforcement learning! No programming skills are needed at all for building and using state-of-the-art machine learning models!
The following machine learning models are supported:
The following machine learning models are supported:
- Supervised Learning - Support Vector Machine Model
- Supervised Learning - Random Forest Classification Model
- Supervised Learning - Feedforward Neural Network Regression Model
- Supervised Learning - Feedforward Neural Network Classification Model
- Supervised Learning - Convolutional Feedforward Neural Network Classification Model
- Unsupervised Learning - KMeans Classification Model
- Unsupervised Learning - MeanShift Classification Model
- Unsupervised Learning - DBScan Classification Model
- Unsupervised Learning - Hierarchical Classification Model
- Reinforcement Learning - Deep Q-Learning (Neural Network)
- Dimensionality Reduction with PCA (Principal Component Analyzes)
- Recommendation with Explicit Feedback (Collaborative Filtering) (CFE)
- Recommendation with Implicit Feedback (Collaborative Filtering) (CFI)
Watch the Training Video's or Download the AI-TOOLKIT.
You can use the built-in machine learning models
separately (training and prediction/inference) or you can build a larger
AI system in which several AI models are working together in a flow.
Working together in a flow means that one machine learning model may use
the output of one or more other machine learning models in a continuous
running AI system.
There are several built-in easy to apply machine learning
model templates which makes building a complex AI model
very easy by just some mouse clicks. You can train and test your
models easily.
You can import any delimited text data file.
You can also import images which will be converted automatically
to machine learning data and saved into the database. If you select the "Automatically Convert Categorical or Text values" option then categorical values will be automatically converted to numbers with one of the following options (based on your choices per column):
There is an easy to use database editor which can be used to view and update all AI-TOOLKIT databases. The database editor and the AI-TOOLKIT both support encrypted databases in case you need to secure your data.
- Integer encoding
- One-hot encoding
- Binary encoding
There is an easy to use database editor which can be used to view and update all AI-TOOLKIT databases. The database editor and the AI-TOOLKIT both support encrypted databases in case you need to secure your data.
Built-in how-to use help with links to training video's and
tasks:
Please note that the information presented here may not be up to date with the last version and is meant only for giving a quick overview of some of the features!
AI-TOOLKIT AI Definition Syntax
The project editor is an easy to use AI-TOOKKIT syntax
colored text editor (specifically developed for the AI-TOOLKIT AI definition
format) in which you can define your machine learning models and AI systems. One
project file may contain several machine learning models, which may or may not be
connected to each other (machine learning flow). The syntax is very simple and
no programming skills are needed. The image below shows one of the example projects, the SVM
Classification Example. Please note that there are several other types of AI
models available such as neural networks, random forest, etc. (see
later).
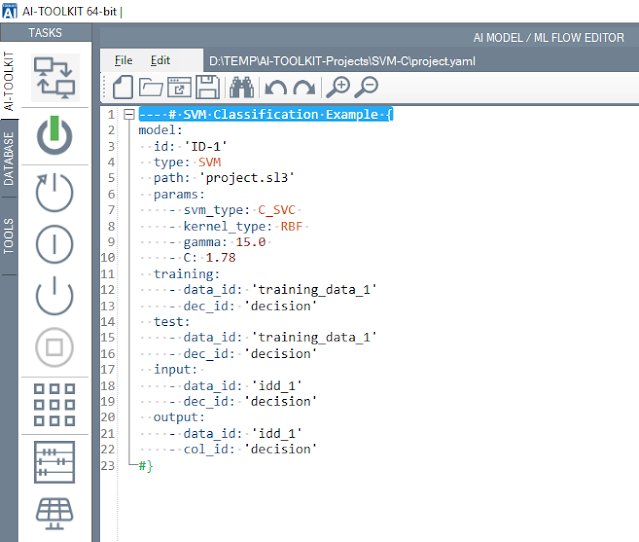
The syntax follows the YAML format with special symbols
defined for the AI-TOOLKIT. Without programming skills you can make
sophisticated machine learning models. There are only a few simple rules (e.g.
the indentation, the use of - (dash) and : (colon), etc.) and pre-defined
symbols such as C_SVC, RBF, etc. Everything is explained in the built-in
help.
Model types and their parameters
With the Insert Template button you can insert a pre-defined
model for each model type into the editor (see above). You only have to change the values of
the parameters or insert some more elements (e.g. neural network layers). This
makes it very easy to make your AI models.
The data for training, testing and prediction is stored in an AI-TOOLKIT database. You can define the database table names with the 'data_id' parameter. The decision variable is defined with 'dec_id'. It is also possible to get the data for an AI model from several database tables (by defining several data_id's).
The Support Vector Machine Model (SVM) (Supervised Learning)
The support vector machine model has several parameters which
can be defined as shown in the former image. This is the SVM classification
model, for a regression model there are some other parameters which are
explained in the built-in help.
There is a built-in SVM Parameter Optimization module. With the parameter optimization module you can automatically determine
the optimal combination of the parameters influencing the SVM model. Depending
on the chosen model (classification or regression) and the Kernel Type there
are different parameters which are being automatically optimized.
The Random Forest Classification Model (RF) (Supervised Learning)
The Random Forest model has also several parameters which can
be defined similarly to the SVM parameters.
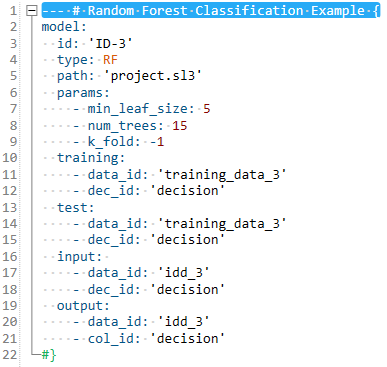
Neural Network Models (regression and classification)
With these models you can build complex feed-forward neural
networks easily. There are many layer types available as for example:
1. Intermediate Connection Layers:
-
Linear
-
LinearNoBias
-
IdentityLayer
-
DropConnect
- etc.
2. Activations Layers:
-
TanHLayer
-
SigmoidLayer
-
ReLULayer
-
LeakyReLU
-
PReLU
-
FlexibleReLU
-
SoftPlusLayer
- etc.
3. Special Intermediate Layers:
-
Dropout
-
BatchNorm (batch normalization)
-
LayerNorm (layer normalization)
- etc.
4. Convolution Layers:
-
Convolution
-
MaxPooling
-
MeanPooling
Building a good convolutional neural network is difficult but the
AI-TOOLKIT has some built-in features which make it easy. Two of these features
are the built-in templates and the Convolution Network Calculator application (see later).
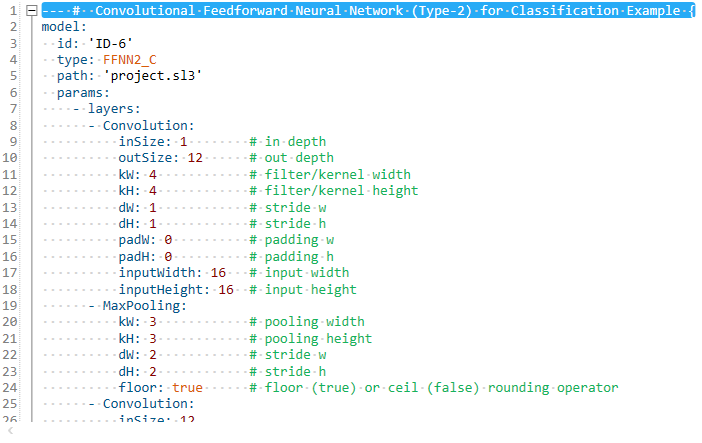
Convolutional Neural Network Calculator App
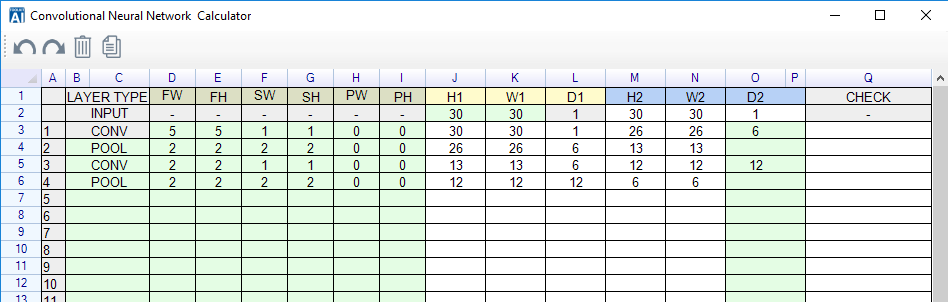
The application is a simple built-in spreadsheet in which you fill in
the green cells and the results automatically appear. When you are ready you can
copy the automatically generated AI-TOOLKIT script (see the code example in the
Convolutional Feedforward Neural Network Classification Model section)
and paste it into the project file. You will need a minimum understanding of convolutional
networks in order to be able to build one, but no programming skills are needed for this!
Unsupervised Learning
This model will help you to classify your data automatically by looking at the relationships and hidden patterns in your data.
Reinforcement Learning
You can build and train your Reinforcement Learning Agent and ask it to do specific tasks. This can be applied in any discipline, research and business field. The AI-TOOLKIT is the first software to allow this without the need for any programming!
Training, Testing and Prediction (Inference) Modules
With these modules you can train, test and predict individual
models defined in the project file. You will be able to select which model you
want to use. The results will be saved directly into the database defined in the
project file (trained models, test results, predictions, etc.).
AI-TOOLKIT Continuous Operation (Auto ML Flow) Module
You can use this module for continuous training and prediction
of several (possibly to each other connected) models. After you push the 'Start
Auto ML Flow' button the software will cycle through all models in the project
file and will train them if necessary. It will check the database for new
prediction requests and will do all open predictions too. All prediction results
will be saved into the database as defined in the project file (input, output).
You can build complex AI systems in which several ML models are working together
(with connected input and output). You can also add data to the database with an
external application while Auto ML Flow is running and in this way connect e.g.
your business processes to an AI system.
The Built-in Database
The AI-TOOLKIT database is a fast built-in SQLite
database with some pre-formatted special tables which are setup automatically by
the software. You do not need to have database skills for using the software.
One of the advantages of using the built-in database is that all of your data is
stored in one place (can be several GB's). You can of course use a separate
database for each of your projects or even per ML model. The database can be encrypted in case you need extra
security.
Built-in Tools
Import Delimited Data Module
With this module you can import any delimited data file into
the AI-TOOLKIT database.
Import Images Module
With this module you can import any image or image collage
(several images in one larger image) into the AI-TOOLKIT database. The images
will be converted automatically to the necessary ML format. The software can automatically extract the classification labels from the file names (if they are defined).
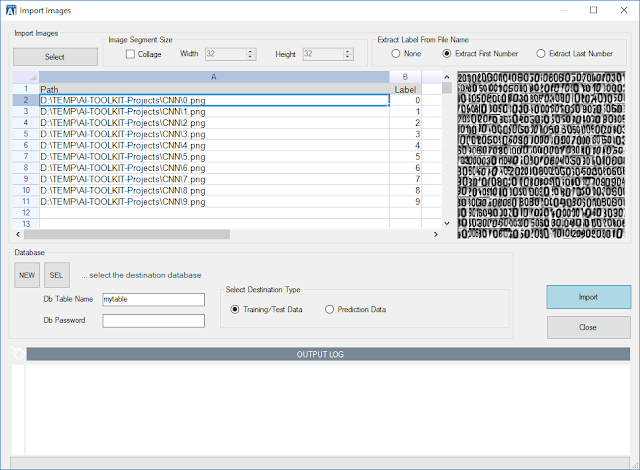
Data Analyzes Module
With this module you can analyze the data defined in the AI-TOOLKIT project
files (for each ML model). The data will be collected exactly as you define it
in the project file and thus it may come from several database tables.
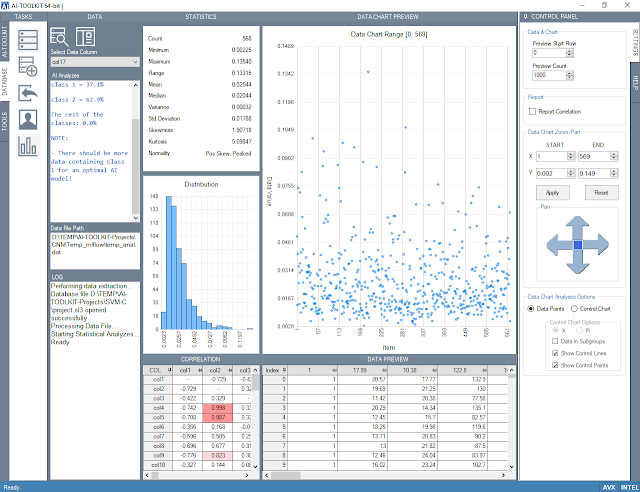
You can also choose to show the Control Chart of the selected column.
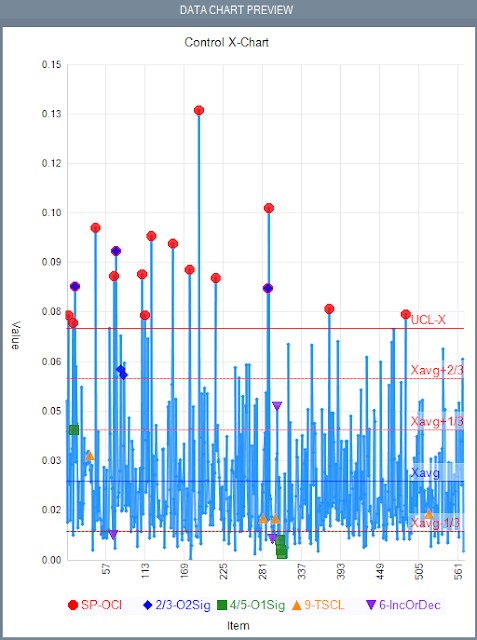
You can select the desired column (Select Data Column) for
which all analyzes results and charts will be shown. The Data Chart Preview and
the Data Table Preview may contain only a subset of your data. You can choose
the subset in the right sidebar (preview start row, preview count). In the correlation table the correlation between all columns
are shown. High correlations are highlighted with darker colors. The Statistics table shows the most important statistics of
the chosen data column. The AI Analyzes short report, on the left side of the screen, summarizes some important aspects of the data quality for AI model training. There are several settings and pan/zoom options in the right
control panel for the main data chart. The blue arrows pan, and in case of the
chart is at the end or beginning of the axis, then also zoom the data chart. In the Data Chart Analyzes Options you can choose to show the
data or a control chart (X or R). Control charts give often very useful extra
information about the data, which may be used e.g. for root cause analyzes of
problems or variation analyzes. The better the quality of your data the better
your AI model will be! You can automatically generate a PDF, MS Word, etc. report
with the Analyzes results by using the built-in Report generator and viewer
(Generate Report button). You can select in the right control panel whether you
want to report the correlation between the data columns. You can also print the
report.
Database Editor
The built-in sophisticated database editor can be used to manual editing or viewing of the AI-TOOLKIT databases. You do not need database
skills for the AI-TOOLKIT but this tool allows you to perform even complex
database queries, encrypt/decrypt the database, edit database tables, etc.
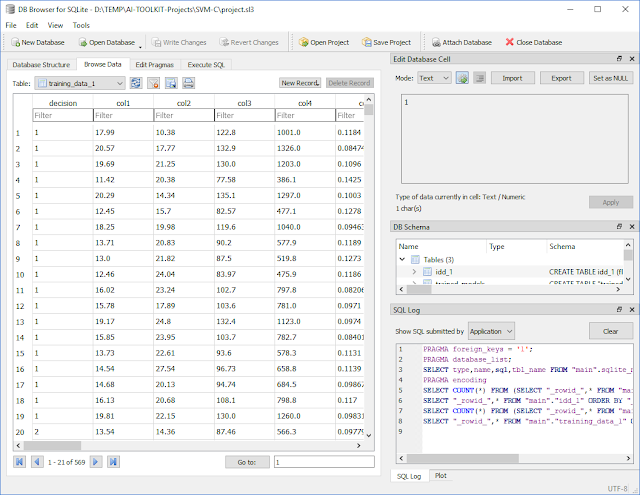
* DB Browser for SQLite - sqlitebrowser.org (c) (GPL/MPL) 2017
Image Editor
The built-in sophisticated image editor can be used to edit
(reduce noise, sharpen, change color, add special effects, etc.) or convert
images (png, jpg, gif, tif, svg, pcx, psd, etc.) and to create an automatic
image collage (several images in one larger image). An image collage can be used
as input to the AI-TOOLKIT and makes the definition of the labels
(classification) easier.
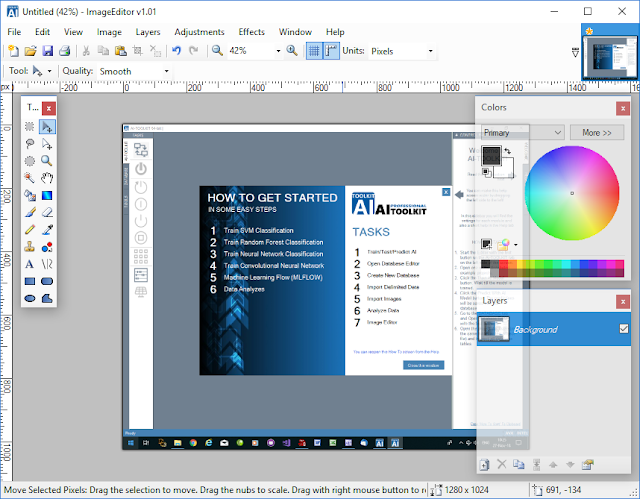
* based on PaintNet - dotPDN LLC (c) (MIT) 2009
DeepAI Educational
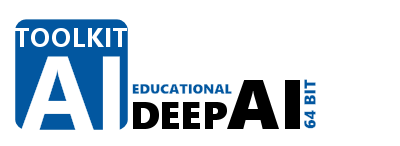
DeepAI Educational is included in the AI-TOOLKIT
distribution. Multi-layered and multi-node deep neural networks made easy
and visible! Despite the fact that deep neural networks modeling is a complex
task DeepAI makes this task simple by intuitively and automatically applying
design patterns to your problem. Even someone without knowledge of neural
networks may train DeepAI successfully. The task of neural network modeling is,
roughly speaking, reduced to just choosing the input functions, the number of
layers and the number of nodes per layer. Each node is automatically connected
to the next layer's nodes and like this the neural network is formed.
This is not a state of the art neural network modeling tool
but rather an educational tool which helps you to understand how deep neural
networks work! If you need a state of the art AI tool then use the AI-TOOLKIT
explained here above.
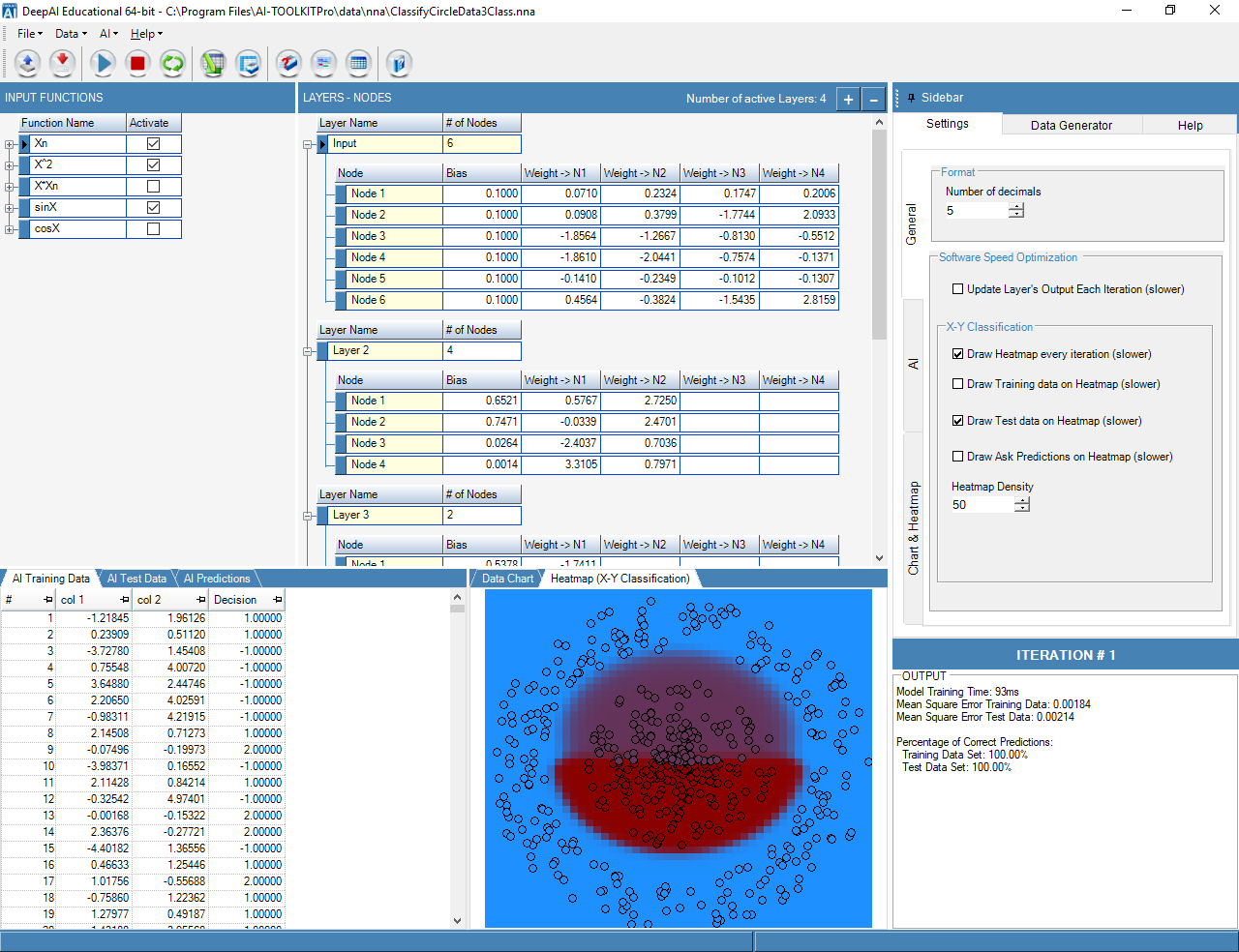
For what can you use the AI-TOOLKIT?
You can use the AI-TOOLKIT for many different purposes. The
possible applications are endless: AI research, Business process improvement,
decision making in several disciplines (management, marketing, finance, etc.),
security applications, knowledge management, illness and cure discovery, R&D in
all disciplines and sectors, making decisions about buying/selling stocks on the
stock exchange, etc.
Read the Knowledge Articles for a lot of application examples!
Business processes can often be modeled with data originating from different steps of the business process.
For example the interaction of the users with the process steps may generate
data at each step. This data can then e.g. be used to make decisions about which
next process step to take. It is also possible that the data is rather complex and contains many variables (many columns of data) and that it would be
beneficial to automate the process step without the intervention of the process
managers. The AI-TOOLKIT can be easily connected to any business process
management (BPM) or ERP software and automate the processes with several trained
AI models. And all of this without the need for programming skills!
AI-TOOLKIT and the AI-TOOLKIT Artificial Intelligence
Engine are (C) Copyright 2016-present Zoltan Somogyi, All Rights Reserved. For
more information and third party copyrights see the software documentation and
the included license agreement in "License.rtf".
Learn about the application of Artificial Intelligence and Machine Learning from the book "The Application of Artificial Intelligence | Step-by-Step Guide from Beginner to Expert", Springer 2020 (~400 pages) (ISBN 978-3-030-60031-0). Unique, understandable view of machine learning using many practical examples. Introduces AI-TOOLKIT, freely available software that allows the reader to test and study the examples in the book. No programming or scripting skills needed! Suitable for self-study by professionals, also useful as a supplementary resource for advanced undergraduate and graduate courses on AI. More information can be found at the Springer website: Springer book: The Application of Artificial Intelligence. |
![]() |